The rise of digital banking has made financial transactions faster and more accessible, but it has also increased the risk of fraud. With cybercriminals constantly evolving their tactics, banks and financial institutions are turning to artificial intelligence (AI) to improve fraud detection and enhance security measures. By using AI-powered systems, banks can identify suspicious patterns in real-time, protect customers, and reduce losses. This article explores how AI is transforming fraud detection in banking, with case studies and insights on the impact of this technology.
The Growing Need for AI in Banking Fraud Detection
Fraud is a serious issue for the banking industry, with global losses estimated to reach over $200 billion by 2025. Traditional fraud detection methods often rely on rule-based systems that can’t keep up with sophisticated attacks. To combat this, many banks are implementing AI-driven systems that can adapt to new fraud patterns, detect anomalies, and respond within milliseconds. Companies like American Express and others are setting a new standard in fraud detection with advanced AI technology, leading to a safer banking experience for consumers worldwide.
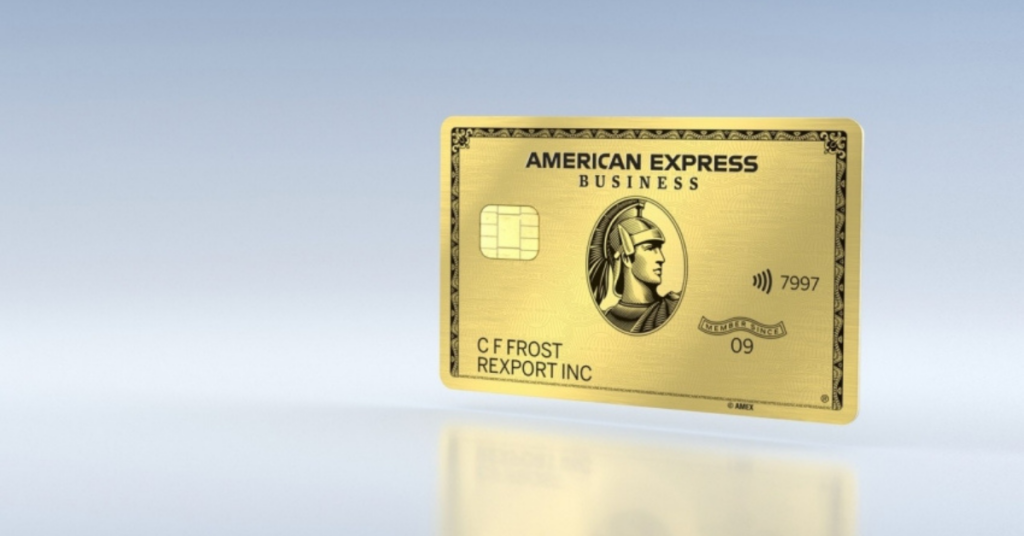
How AI Detects and Prevents Fraud in Real-Time
AI-based fraud detection uses various techniques to identify suspicious behavior and stop fraud before it affects customers. Here are some of the most common AI methods used in banking:
1. Machine Learning Models
Machine learning enables banks to analyze vast amounts of transaction data and recognize patterns that might indicate fraud. For example, if a customer in New York suddenly has transactions occurring in multiple countries within hours, the system can flag this as suspicious activity. American Express, for instance, leverages long short-term memory (LSTM) models using NVIDIA DGX™ systems to analyze transaction patterns and detect fraud with greater accuracy and efficiency.
2. Deep Learning Networks
Deep learning networks, such as convolutional neural networks (CNNs) and recurrent neural networks (RNNs), enhance fraud detection by processing complex data sets. These models can detect hidden patterns in data that traditional rule-based systems miss. The speed of these models enables banks to analyze transactions within milliseconds, ensuring that suspicious activity is identified before any harm is done.
3. Natural Language Processing (NLP)
Natural Language Processing (NLP) helps banks process unstructured data like emails, social media messages, and chat logs to identify potential fraud or phishing attempts. For instance, NLP can identify keywords or phrasing commonly used in phishing schemes, flagging messages before they reach customers.
AI-Powered Tools Leading the Way in Banking Security
Advanced AI tools and platforms are helping banks take fraud detection to the next level. Notable technologies include:
- NVIDIA TensorRT™: Optimizes AI models for real-time inference, improving the speed of fraud detection and minimizing latency.
- Microsoft Azure AI and Google Cloud AI: Cloud-based platforms that support AI-powered fraud detection systems for scalability and accessibility.
- Triton™ Inference Server: NVIDIA’s scalable server allows banks to deploy AI models quickly and efficiently, enhancing response times for fraud detection.
For instance, American Express uses Triton™ Inference Server to deploy its AI fraud detection models, resulting in a 50x improvement in throughput over CPU-based systems and allowing for near-instant responses to suspicious activities.
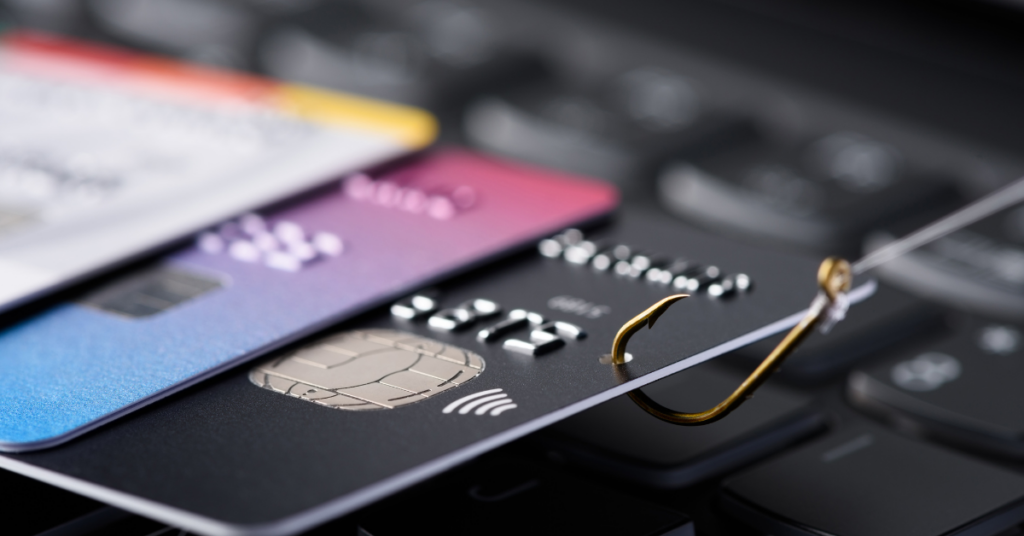
Benefits of AI in Fraud Detection for Banks and Customers
The integration of AI into fraud detection has profound benefits for both banks and customers, offering a secure and streamlined financial experience:
Improved Accuracy and Reduced False Positives
AI reduces the number of false positives, which are common in traditional fraud detection systems. By analyzing customer behavior and transaction histories, AI models can distinguish between actual fraud and unusual but legitimate activities, creating a smoother experience for users and preventing unnecessary transaction freezes.
Real-Time Protection for Customers
AI systems work within milliseconds, quickly identifying and stopping suspicious transactions before they are completed. Real-time fraud detection not only protects customers but also prevents extensive losses for banks, creating a safer banking environment.
Scalability and Adaptability
AI-driven fraud detection solutions can scale with an institution’s needs, whether for local banks or large financial networks. As fraud tactics evolve, AI models can adapt and learn new patterns, staying ahead of cybercriminals without frequent manual updates.
Global Trends in AI-Powered Fraud Detection
AI-powered fraud detection is quickly becoming a global standard, with many countries adopting these systems to protect their banking infrastructure. Here’s a look at how some regions are leading the way:
- United States: Many U.S. banks use AI for fraud detection, including major players like JPMorgan Chase and Citibank, which utilize both machine learning and cloud-based AI solutions to monitor transactions.
- Europe: European banks, such as BNP Paribas and Barclays, are using AI to comply with stringent security regulations and safeguard customer data. AI-driven fraud detection helps these institutions meet EU security standards like PSD2.
- Asia-Pacific: In regions like Singapore and Japan, AI-driven fraud detection has become crucial due to the rapid digitization of financial services. Banks in these countries are investing heavily in AI infrastructure to ensure customer trust and prevent cybercrime.
Challenges in Implementing AI-Based Fraud Detection
While AI offers numerous advantages, implementing these systems comes with challenges:
Data Privacy and Regulatory Compliance
Banks must navigate data privacy laws, such as the GDPR in Europe, which restrict data usage. AI models require extensive data to detect fraud accurately, so institutions must balance these needs with legal compliance to protect user privacy.
Integration with Legacy Systems
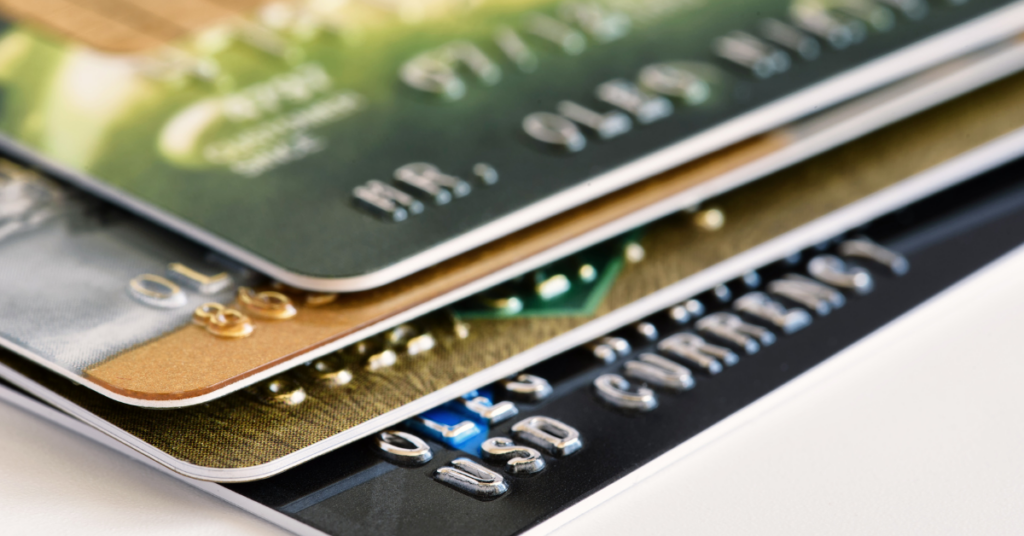
Many banks still operate on legacy infrastructure that may not be compatible with modern AI models. Integrating AI-based fraud detection into these systems requires substantial time and investment, which can be a barrier for smaller institutions.
Model Transparency
AI models, particularly deep learning networks, are often complex and can act as “black boxes” with limited interpretability. Banks must ensure their AI systems are explainable, so they can understand and justify fraud detection decisions when needed.
The Future of AI in Banking Security
AI’s role in banking security is expected to grow, with continuous advancements in machine learning and deep learning models. Future AI applications may involve predictive analytics to forecast potential fraud hotspots and the use of blockchain for additional transaction security. AI’s ongoing development promises more robust defenses against cybercrime, setting the stage for a safer, more resilient financial ecosystem.
Conclusion
AI is revolutionizing fraud detection in banking by offering faster, more accurate solutions that protect customers and financial institutions alike. Through machine learning, real-time monitoring, and innovative platforms like NVIDIA’s Triton™ Inference Server, banks are setting new standards for security. As AI technology advances, the banking industry can look forward to even more sophisticated tools to fight cybercrime and ensure secure digital transactions.
For more insights on AI-driven innovations in finance and beyond, visit Chloroville.